5 Reasons Business Leaders Should Think Twice About ChatGPT
AI has its place in the modern enterprise, but large language models suffer a lack of context, clarity, and accountability
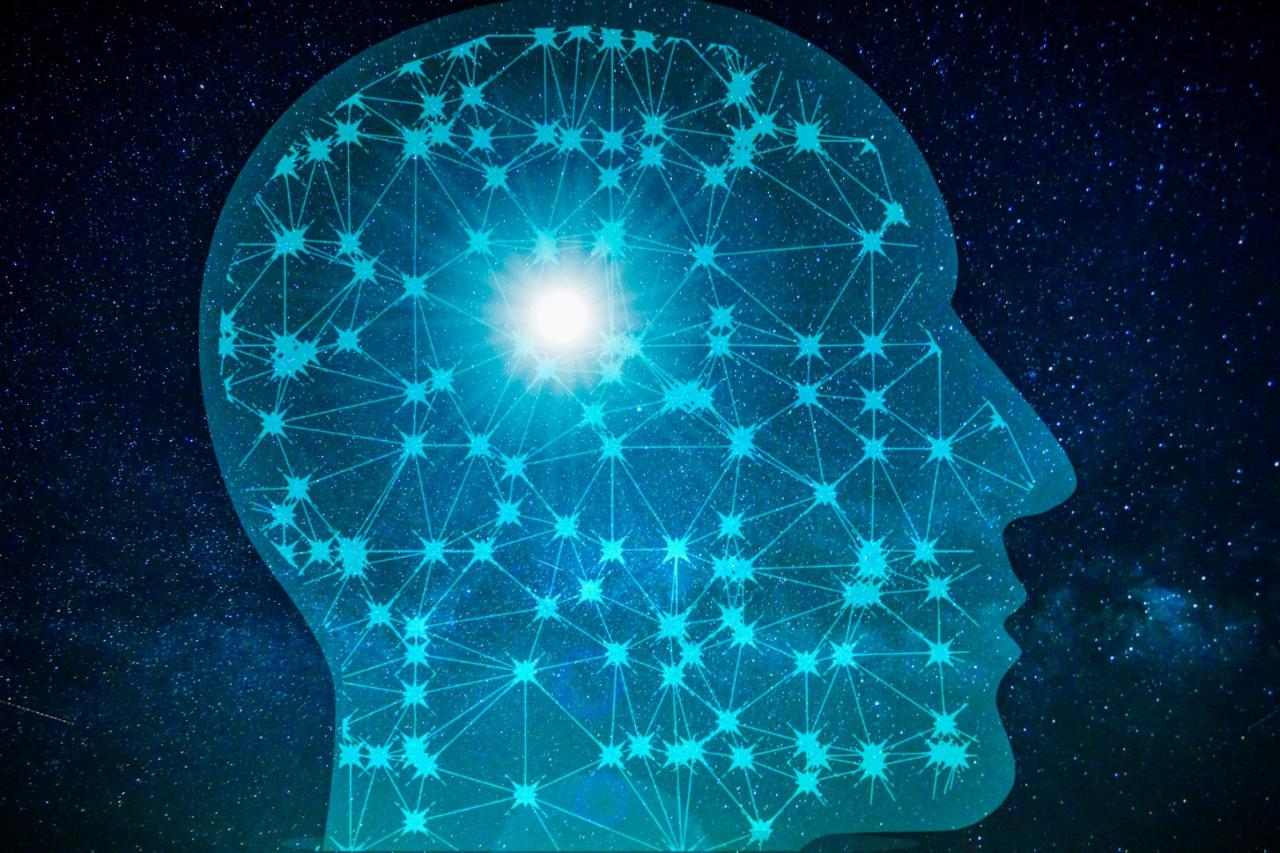
Since it was first released, OpenAI’s ChatGPT has taken the world by storm, gaining more than 100 million users in the first two months. This is unsurprising, given the seemingly savant-like capabilities the so-called generative AI has demonstrated. The model has been shown to be proficient at answering complex questions, summarizing vast volumes of text, solving simple math problems, generating valid computer code, and even writing original prose and music. Encouraged by its reception, Microsoft immediately deployed similar technology to its Bing search engine, and to Microsoft 365 shortly thereafter.
Not to be outdone, Google and Baidu quickly followed up by announcing their own AI chatbots. But their releases have been much rockier, to say the least. The launch of Google’s “Bard” went disastrously wrong when Bard “hallucinated,” making a simple factual error in its first demonstration. This cost Google its momentum against Microsoft and OpenAI, as well as $100 billion in share value. Perhaps learning from this, Baidu chose to take a more conservative approach when announcing their “Ernie” model by prerecording much of the presentation and removing the potential for unforced errors. However, this lack of spontaneity was not well received.
Google and Baidu’s experiences point to a serious problem with the accuracy of large language models (LLMs) — something ChatGPT is not immune to. But inaccuracies are just one of several factors business leaders must consider before signing their companies up for the AI chatbot revolution.
Reason 1: There’s no clear use case
Why has Microsoft incorporated chatbot technology across all its most popular tools? The new assistant, named Copilot, is apparently going to "fundamentally change the way we work" by summarizing meetings, creating PowerPoint presentations, drafting emails, and generating charts and graphs in Excel. However, Microsoft cautions that the technology can still be “usefully wrong.”
The goal with this technology is to limit the busywork, allowing employees to focus on the “20% of work that really matters.” But if Copilot sometimes gets the busywork wrong, doesn’t it create more work to proof and correct?
The truth is, Microsoft is throwing this technology at every use case to see where it sticks. There is no singular purpose for large language models, and that’s a problem. Instead of training an AI model to do one thing exceptionally well — reading medical scans, for example — LLMs are the quintessential Jacks-of-all-trades and masters of none.
OpenAI readily admits that ChatGPT “sometimes writes plausible-sounding but incorrect or nonsensical answers.” This disqualifies the chatbot from many business use cases. You can’t have a chatbot giving customers the wrong information, incorrectly diagnosing a medical condition, deciding who should or should not be granted a loan, or writing nonsense content for your website. And, as OpenAI acknowledges, there’s no simple fix for ChatGPT’s rampant inaccuracy. That’s because of the data it ingests.
Reason 2: They’re fed on junk data
Large language models need data. Lots of it. And because they’re so big, and so expensive to run, that data needs to be cheap. The cheapest data is whatever’s available on the internet — billions of pages of content from the Wall Street Journal, BBC, Associated Press, Reuters… and The Onion, BuzzFeed, and your crazy uncle’s blog.
How does a machine differentiate between a peer-reviewed study, backed by gold-standard experimental research and published on PubMed, and a conspiracy theory concocted by your aforementioned crazy uncle and posted in an unhinged Wikipedia edit? It can’t. Particularly when misinformation so often mimics the markers of authenticity in order to confuse human readers.
Any AI is only as good as the data it ingests. To serve a specific use case, data must be clean, highly curated, and originate from the domain to which the resultant model will be applied. To return to the medical scan case, for example, there’s no use asking a chatbot to scrape every image it can find from the internet and then reliably identify cancer on an MRI. The AI has no frame of reference for accuracy, no logic to differentiate between fact and fiction. Chatbots are simply probabilistic models that predict the next word. They’re driven by data, not facts.
Reason 3: They can’t handle the truth
We’ve all seen enough movies to know that artificial intelligence doesn’t have an objective knowledge of right and wrong, truth and lies. AI chatbots only know what they have been trained to know, but even the smartest chatbot doesn’t “know” things in the same way a human does. That means it doesn’t question what it is learning, making it alarmingly easy to introduce bias.
With narrow models, bias can be considered and addressed from the outset. With LLMs, it’s like playing a constant game of whack-a-mole. Because they’re constantly learning from user feedback, and probabilistic models can only parrot information based on the data they have ingested, they can be easily manipulated — and some users will always try to trick the machine.
Faced with these challenges, it’s unreasonable to think LLMs will ever get their responses 100% right. They’re not search engines and have no way of independently verifying the accuracy or bias of the information they ingest and repeat. As OpenAI admits, “there’s currently no source of truth.” When paired with technology designed to always provide an answer, end users must do extra work to understand what the AI doesn’t know it doesn’t know.
Reason 4: Explainability is impossible
Another problem with large language models is they can’t justify the answers they give. At Aware, we’ve trained our sentiment models on a highly curated set of data labeled with a strict set of rules that define what we consider positive and negative. Similarly, in our toxic speech model, the definitions of what is inappropriate, offensive, or hateful are based on academic and industry research as well as feedback from customers. When our sentiment model scores a sentence, we can point to the exact rules that determined that score. The end user might disagree with an assessment — what constitutes inappropriate language in one workplace might be perfectly acceptable in another, for instance — but the why behind the result is known and can be objectively assessed.
In large language models, trained on massive amounts of Internet content, such explainability is lacking. ChatGPT will be happy to provide the sentiment or inappropriateness of whatever text you provide, and it often does a good job, but how do we know what it defines as positive sentiment or language inappropriate for work? Because the input data are not curated, there is no way to reference the source of these definitions.
While this example may seem trivial, a lack of explainability is essential in highly regulated industries, such as healthcare and financial services. A doctor won’t defer to AI if they can’t understand why the computer made the recommendation it did. To be truly useful, chatbots have to both be right, and explain their reasoning.
Of course, it’s always possible to use an LLM like ChatGPT as a foundation for a model that is then “fine tuned” to the task at hand, but there are at least two significant issues with this. First, it’s not easy (or even possible?) to know how much of the baseline influences the final model. With tens of billions of parameters to adjust, there is a lot of baggage to overcome in order to make sure the end result is as intended. Second, fine tuning an LLM is likely to be much more expensive than training and deploying a “narrow” model that answers a single, well-defined problem.
Reason 5: They put your data at risk
Large language models are always learning, and they’re using your data to do it. OpenAI is fully transparent that “Your conversations may be reviewed by our AI trainers to improve our systems.” That means any time an employee enters business-sensitive information and asks the chatbot to generate an email, press release, blog post, or presentation, that data becomes part of the fabric of the AI and can be returned as a result to any other user.
Businesses in highly regulated industries, or in competitive industries where disclosing intellectual property could spell the end of a company, should pay attention. Once a company deploys a tool for employees to use to do their work, they must anticipate sensitive data being entered into that tool. It’s something we’ve seen time and again at Aware — check out the time we uncovered thousands of PCI details in one organization’s Slack environment. If your business owns data you don’t want to become public, or leaked to a competitor, you need to think twice about authorizing the use of any third-party large language models in your workplace.
Final thoughts
Artificial intelligence has the potential for changing the way we do business forever, but only when it is used correctly. That means identifying specific use cases, curating your data, and giving the AI clear rules to follow under the hood. Artificial intelligence may have higher learning capacity, but it cannot replicate human reason or logic.
Before embracing ChatGPT or other large language models, business leaders must understand the costs, pitfalls, and risks associated with this kind of technology, and not create a situation where they end up depending on something they don’t understand.
Dr. Jason Morgan is Vice President, Behavioral Intelligence (Data Science, Data & ML Engineering) @ Aware: The Voice of Innovation